Machine learning could help predict defects, fraud and cancer
Machine learning could help predict defects, fraud and cancer
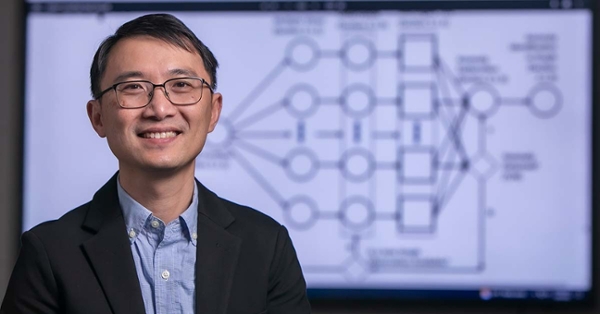
When Dr. Shen-Shyang Ho looks at a graph, he sees more than abstract data points. In the dynamic graphs he studies, the computer science researcher sees complex networks that change over time.
An associate professor in the Department of Computer Science in Rowan University’s College of Science & Mathematics, Ho has studied and developed machine-learning technologies for detecting anomalies in various application domains for nearly 20 years.
“Anomalies are deviations from the normal,” explained Ho, who also coordinates Rowan’s master’s degree program in computer science. Many anomalies are undesirable, he added, such as financial fraud, suspicious behavior, manufacturing defects and abnormal findings on medical tests.
Previously, Ho developed novel approaches for identifying meaningful anomalies from images containing defects in turbine blades or cancerous cells. With a new $273,047 grant from the National Science Foundation, he will spend the next three years focusing on developing new approaches for detecting, explaining and predicting anomalies in dynamic graphs that continuously evolve.
“When you detect an anomaly, the next stage is decision-making,” Ho said. “In dynamic graphs, the earlier you can detect or predict anomalies, the better, because you can start mitigation efforts. If the anomaly isn’t detected until later, you might not have time.”
Anomaly detection through machine learning offers opportunities to stop credit card fraud, provide early cancer treatments or correct manufacturing defects. Deviations in an aircraft flight pattern, for example, might indicate that a plane is facing a crisis, such as a hijacking.
Discovering the rapid spread of an infectious virus across networks of international airports by detecting anomalies in dynamic graphs representing cross-country human movements allows experts to implement policies to contain the spread of disease.
Dynamic graphs representing a power grid with substations, transformers and homes can help utility companies understand and detect abnormal usage patterns, so they can better advise consumers on precautions they can take to prevent an outage.
The benefits of data-driven approaches to detect and predict anomalies have fostered an interest in machine learning technologies across numerous industries. Early detection reduces financial costs, while preemptively identifying public health concerns, disease progression, manufacturing defects and aircraft crises can save lives.
Existing approaches to machine learning offer general solutions for anomaly detection, but “good solutions are problem-specific,” Ho said. “Our approach of looking at specific problems from multiple views extracted from the dynamic graph data allows us to explain why we make the call that an anomaly is going to occur in the near future.”
“There’s always a tradeoff between false positives and false negatives,” Ho added. “If a false positive triggers an alarm in your anomaly detection system, your mitigation efforts are wasting resources. On the other hand, a false negative misses the detection of an anomaly which could have catastrophic consequences. Our proposed approach allows one to choose the amount of false positives he/she can tolerate before the predictive model is even used.”
“In fact, based on our proposed approach,” Ho said, “I can tell you whether there will be an anomaly in the near future, determine how likely it is going to happen, and explain why I’m making that prediction.”